Generative AI for business applications is a hot topic but comes with a certain level of risk. Imagine investing months into an AI project only to see it perform poorly, receive bad feedback, and leave stakeholders thinking it’s too expensive or not ready for production. The waste of resources, frustrated team, and missed opportunities can be devastating. But it doesn’t have to be this way.
Generative AI for Business: 12 steps to success
The key is following a proven framework that addresses these challenges head-on and implementing that framework from the start. So, let’s break down our 12 steps for running a successful generative AI project, based on Google’s white paper, “The Executive’s Guide to AI.”
Step 1: Identify a Specific Problem
First things first, identify a specific problem your generative AI project can tackle. It could be anything from speeding up customer service response times to automating data analysis. The key is to focus on one challenge that will significantly impact your business. Delve deep into why it’s a challenge by employing the “Five Whys” technique, asking why something is a challenge five times to get to the root of the issue. Prioritising a challenge gives your project a clear goal and direction, making it easier to measure success and allocate resources effectively.
Step 2: Choose a Target User or Persona
Next, choose a target user or persona for your AI solution. This could be a customer, an employee, or a specific department. Selecting a persona helps tailor the AI solution to meet the nuanced and highly specific needs of that user. Once you’ve got your persona, define key performance indicators (KPIs) to measure the success of your project. These KPIs will provide measurable goals to track progress and impact, helping the project achieve further funding and inspire additional initiatives in the future.
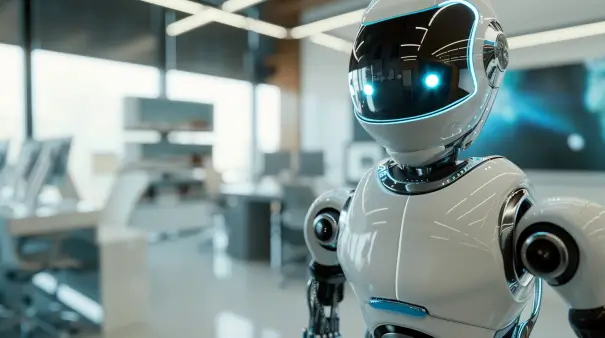
Step 3: Define Key Performance Indicators (KPIs)
When choosing KPIs, focus on setting specific ones for your project. For example, if you’re building an AI agent that lets you chat with your documents, a KPI could be how quickly you can locate information compared to traditional methods. There are also several generic KPIs that can fit most projects, but we’ll circle back to those later.
Step 4: Brainstorm Potential Solutions
Get your team together for brainstorming sessions to explore potential solutions. Use the “How Could We” approach to encourage creative thinking and collaboration. This step fosters innovation and ensures that multiple perspectives are considered, leading to more robust and effective solutions. Asking “How Could We” helps you make the solution better by thinking about additional features or how the user could use the solution in new ways.
Step 5: Identify and Gather Data
Identify and gather the data you’ll need for your AI project. This could include internal data, third-party data, or publicly available datasets. Ensure that the data is clean, relevant, and of high quality. Quality data is key to the success of any AI project. Properly sourced and prepared data ensures the accuracy and reliability of your AI model. This should also help you identify which projects to prioritise, as you’ll have good data on some projects and not others.
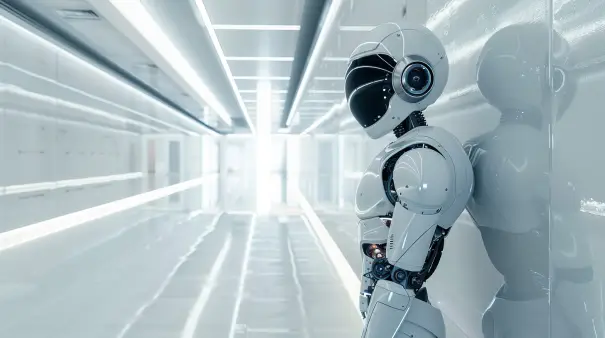
Step 6: Assemble a Cross-Functional Team
Assemble a cross-functional team with diverse skills, including engineers, data scientists, UX designers, and project managers. This “tiger team” will help drive the project forward. A well-rounded team brings together different expertise and perspectives, increasing the likelihood of the project’s success.
Step 7: Develop Prompts
Develop prompts, maybe in a Colab notebook, to test your ideas quickly and efficiently. These prompts should be designed to validate the feasibility and potential impact of your AI solution. Trialling prompts beforehand allows you to test and iterate on your ideas before committing significant resources to the project, reducing risk and ensuring the final solution is likely to be effective.
Step 8: Design a User-Friendly Interface
Design a user-friendly interface for your AI solution, focusing on creating an intuitive and engaging user experience that meets the needs of your target persona. A great UI and UX can make or break your project, as an intuitive interface ensures that users can easily interact with and benefit from the AI solution.
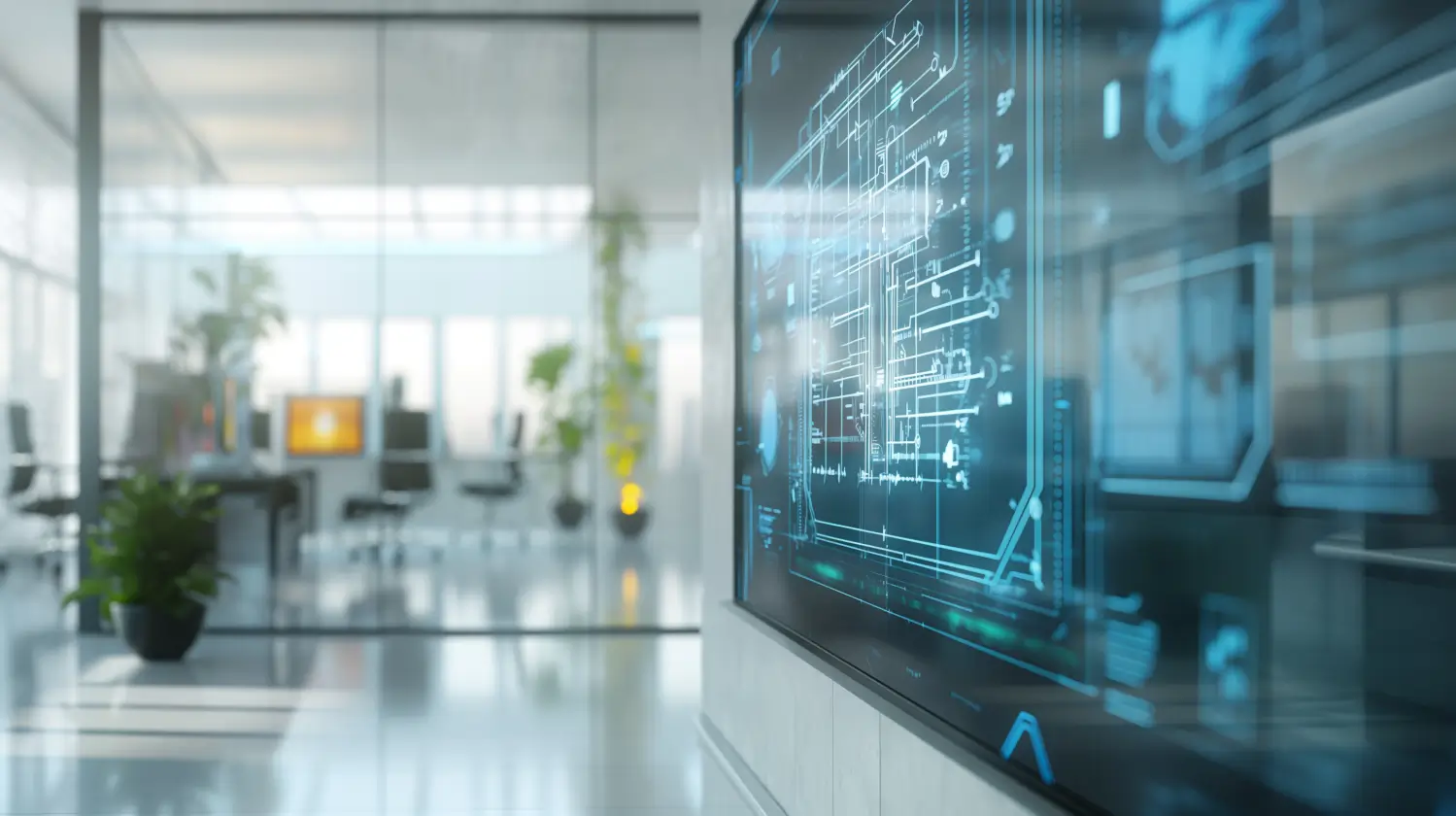
Step 9: Develop a Plan for MLOps
Develop a plan for managing and maintaining your language model, commonly known as MLOps. This includes monitoring performance, making updates, and retraining on new data and feedback. Ensuring the model continues to meet your KPIs is crucial for long-term success.
Step 10: Roll Out the AI Solution
Hopefully, at this point, you’ve proven a successful proof of concept (POC) of your Generative AI tool for your business. Roll out the AI solution to a broader audience. This could include scaling up the infrastructure, providing training, and gathering feedback from new users. Expanding to more users allows you to maximise the impact of your AI solution and gather more data for continuous improvement.
Step 11: Regularly Assess KPIs
Regularly assess your KPIs to ensure that your AI project is on track. Use these assessments to identify areas for improvement and make the necessary adjustments. Ongoing KPI assessments provide valuable insights into the performance and impact of your AI solution, helping you stay aligned with your goals.
Step 12: Launch and Maintain
Finally, launch your AI project into production and establish a maintenance plan to keep it running smoothly. This includes monitoring the infrastructure, performance, addressing issues, and making updates as needed. A successful production launch and ongoing maintenance ensure that your AI solution continues to deliver value over time.
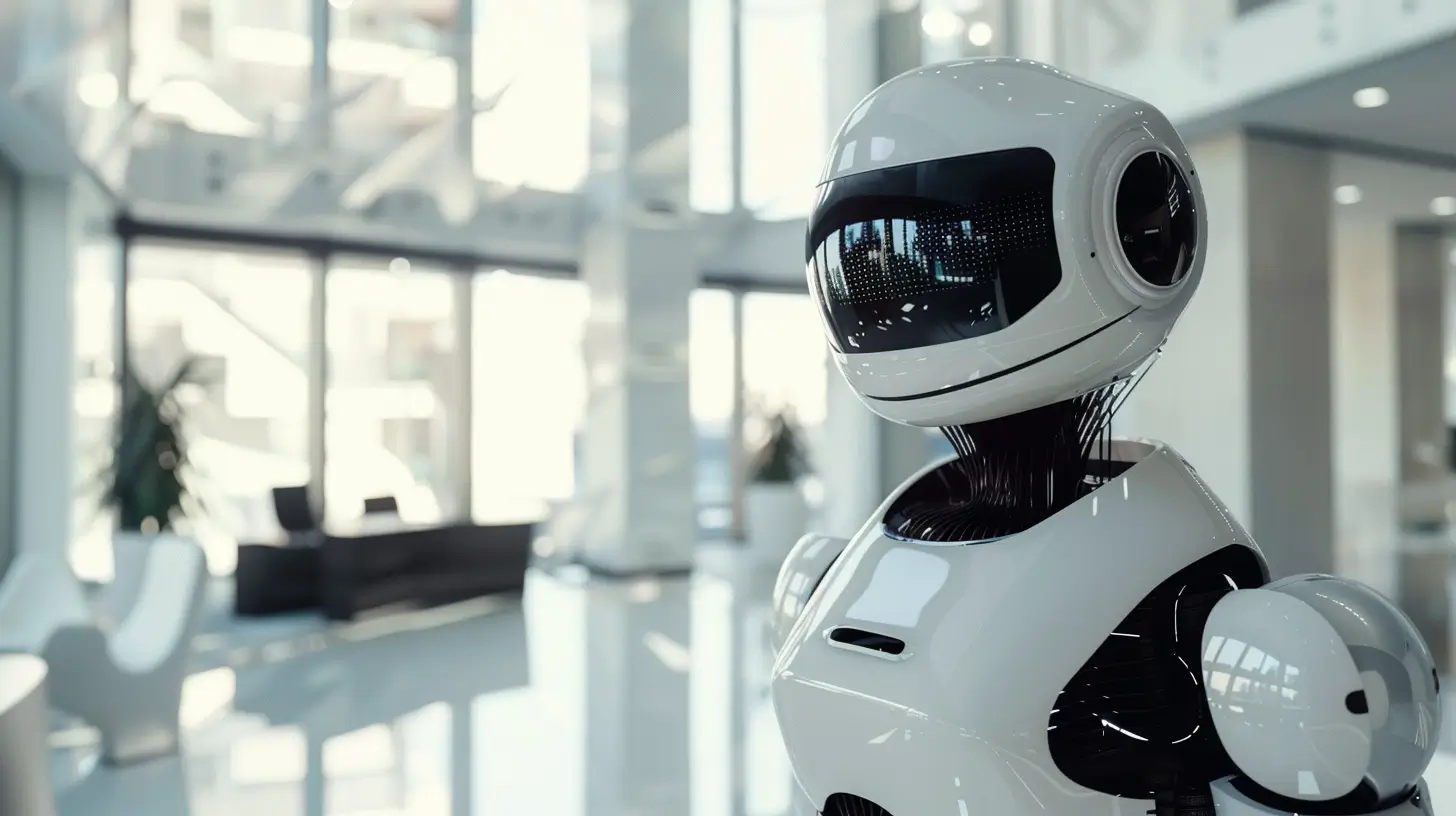
Generative AI for Business: Key Performance Indicators (KPIs)
Now, let’s dive into those KPIs, as they are one of the most critical factors in ensuring your project is seen as a success.
- Accuracy: Measure how accurately your generative AI model produces relevant and correct outputs. Use metrics like precision, recall, F1 score, or mean squared error, depending on your specific use case. High accuracy means your AI model delivers reliable and relevant results, crucial for building user trust and satisfaction.
- Productivity: Evaluate the impact of generative AI on the productivity of your target persona or department. Look at metrics like the number of tasks completed per unit of time, response time, or reduction in manual effort. Boosted productivity showcases the efficiency and effectiveness of your AI solution, leading to cost savings and better use of your team members’ time.
- Customer Satisfaction Scores: If your generative AI use case involves customer-facing applications, use customer satisfaction surveys or feedback to gauge how well the AI system meets customer needs and expectations. High customer satisfaction ensures that your AI solution is hitting the mark, providing a positive experience that can increase loyalty and retention.
- Cost Savings: Measure the business’ cost savings achieved through the use of generative AI. Compare the costs of employing the AI system to the expenses associated with traditional manual processes or outsourcing. Demonstrating cost savings highlights the financial benefits of your AI solution, making it easier to justify the investment.
- Turnaround Times: Evaluate the time taken for the generative AI model to generate responses or outputs compared to traditional business methods. Faster turnaround times can lead to increased efficiency and improved customer experience. Reduced turnaround time showcases the speed and efficiency of your AI solution, which can be a significant competitive advantage.
- Quality of Output: Assess the quality of the generative AI outputs against predefined criteria. Depending on the use case, this can be done through manual review or automated quality checks. High-quality outputs ensure your AI solution meets the required standards and delivers value to your users.
- Error Rate: Quantify the rate at which the generative AI model produces incorrect or undesirable outputs. Minimising error rates is crucial for maintaining accuracy and reliability. Low error rates indicate a robust and reliable AI model, essential for user trust and satisfaction.
- Business Impact: Identify specific relevant business metrics directly impacted by the generative AI use case, such as increased sales, reduced customer complaints, or improved employee retention. Demonstrating a positive business impact highlights the strategic value of your AI solution and its contribution to overall business goals.
- Training Time and Cost: Measure the time and resources required to train and fine-tune the generative AI model. Efficient training processes can lead to faster implementation and quicker time to value. Reducing training time and cost makes your AI solution more accessible and cost-effective, accelerating the return on investment.
- Human-in-the-Loop Metrics: If human intervention is involved in the generative AI process, track metrics related to the efficiency and effectiveness of human oversight. Effective human-in-the-loop processes ensure that your AI solution benefits from human expertise while maintaining efficiency.
- Scalability: Assess how well the generative AI model scales to accommodate increased usage or high demands. Scalability is essential for long-term success. A scalable AI solution can grow with your business, ensuring it continues to deliver value as demand increases.
- Regulatory Compliance: For sensitive domains like healthcare or finance, monitor how well the generative AI system adheres to relevant regulatory requirements and data privacy standards. This protects your business from legal risks and builds trust with users and stakeholders.
With your 12-step project plan and KPIs in place, you can guarantee a better, more measurable outcome for your generative AI project. However, the key is to iterate over time and enhance your solution as you progress through your AI-powered journey.
If you need any assistance or want to learn more about our one-day Data and AI Discovery Workshop, feel free to reach out to the team at Firney. Happy innovating!